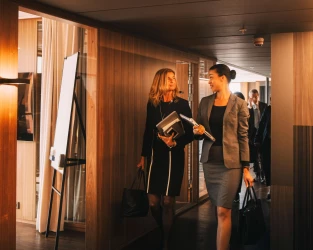
BCG SPOTLIGHT
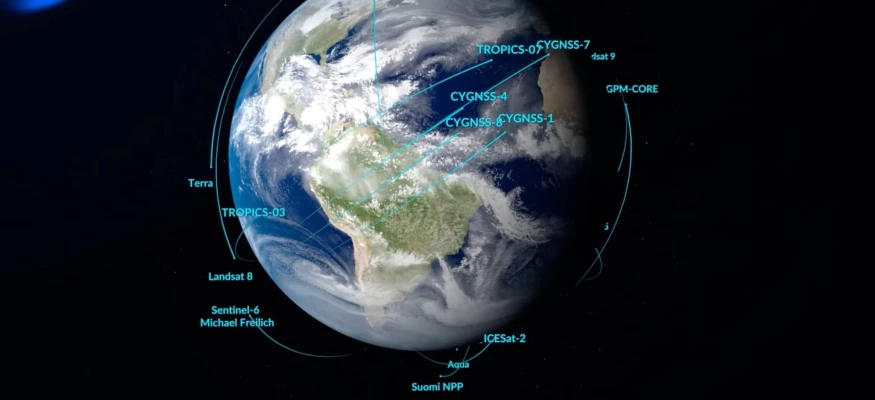
BCG Announces Collaborations with NASA and USRA
We are privileged to work with NASA’s Ames Research Center and the Universities Space Research Association (USRA) to launch a GenAI lab for science and engineering. Harnessing the talents of innovators with decades of experience advancing the state-of-the-art in AI, we are pioneering the critical research and development necessary to propel new Earth science technologies forward—and take our global impact to new heights.
LEARN MORE
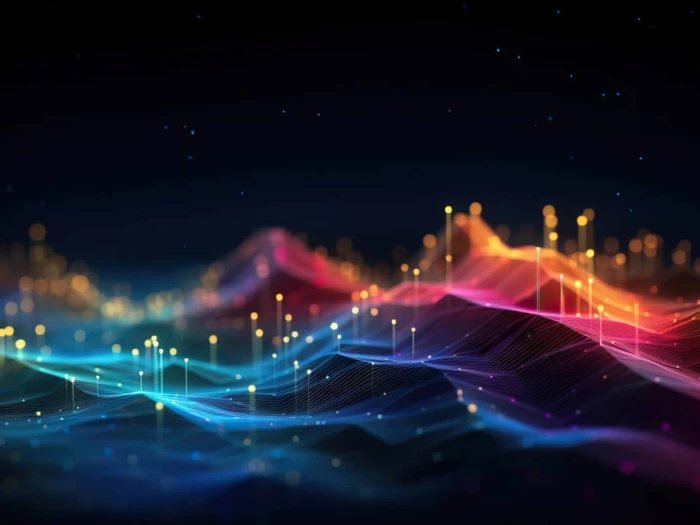
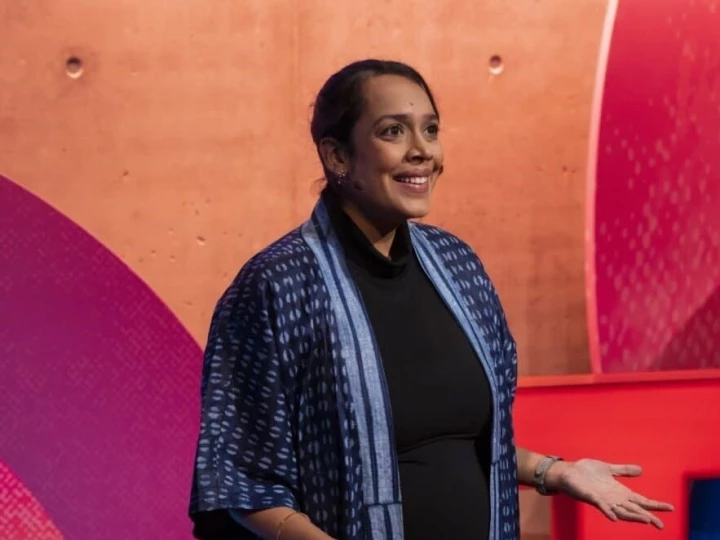
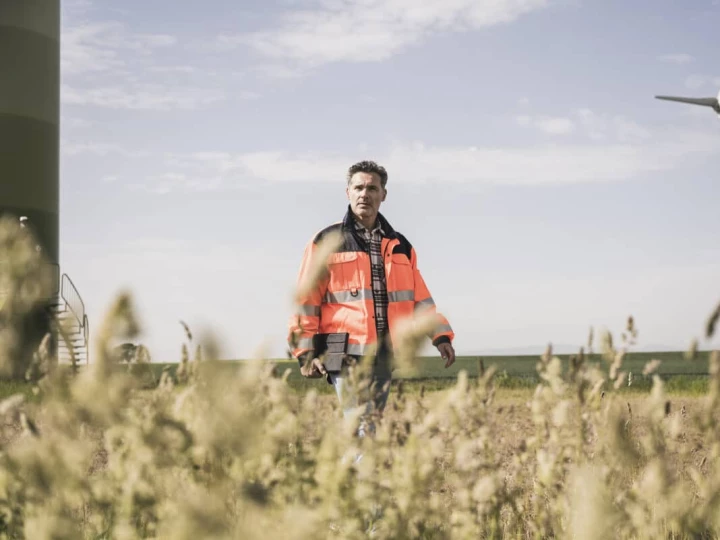
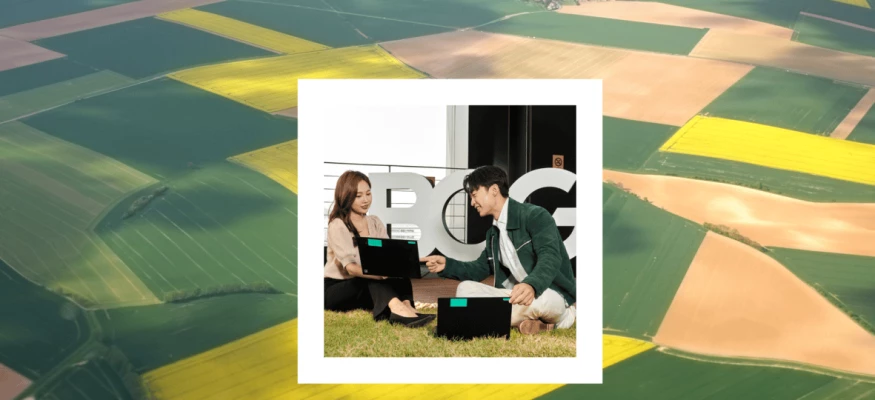
BCG Careers
Go Beyond the Expected
We’re dedicated to helping our clients do amazing things and unlocking the potential of those who advance the world. Join us, and you can too.
APPLY NOW